Implementing optimization projects successfully
Do you have a specific business objective in sight? Maybe it is to lower costs, increase efficiency or design the best supply chain possible? Mathematical optimization is the tool to go for when it comes to making the optimal decision out of billions of theoretical options.
It would only be logical to assume that optimization projects are ubiquitous, yet this is not the case. Why? Well, very promising optimization projects often fail because of how they are implemented. Today, we want to show you how you can successfully put optimization projects into practice and avoid pitfalls.
The project flow: what are the pitfalls?
When viewing the ideal typical flow of a project there are, in our experience, three classic stages where pitfalls lie: At the end of the discovery phase, then when the prototype is translated into reality and its launch in the organization.

The discovery phase: from the idea to the business case
It is important to define the objectives at the start of any project. This mostly entails removing the specific pain points (e.g. costs that are too high) or gaining improvements (e.g. increasing efficiency). This is where the first pitfall lies in wait: If the objectives have only been vaguely defined, their limits merge with similar objectives which do not actually have anything to do with the project. A project with unclearly defined objectives is doomed to failure right from the onset.
Once the objectives have been defined, you can start and come up with ideas on how to implement them. While collecting these ideas usually runs without any major complications, the phase after this is even more critical. Because now you have to derive specific business cases from this abundance of ideas, which can help you to achieve the objectives. As was the case with defining the objectives, the biggest risk lies in the inaccuracy of the business cases. If the work is not performed with the necessary precision, then the cases cannot withstand the loading test with real data.
Let’s take a look at these loading tests. This stage is about first quantifying the potential of the ideas that have been developed within the framework of the business cases. What would be the effects of the idea if we implemented it? How resilient are the assumptions? If there are too many inaccuracies in the first two points, then there is a high probability that the project will not survive the discovery phase.
Prototyping: Taking a short breather in-between
If an optimization project has withstood the discovery phase, then nothing should stand in the way of developing the prototype. In this phase you formalize the ideas that have been developed and transfer them into a practice-based optimization model on the basis of the business cases and often work with sample test data in order to test the functionality of the model. This phase is relatively uncritical, not least because businesses can often get optimization experts, such as OPTANO, on board.
Operationalization: Confronting reality
Once you have a prototype, the next step is operationalization. To be specific, this means integrating the new software into the existing IT infrastructure within the context of productive operation. After this, all the functions are added which offer the finishing touches in day-to-day use and take all the final special cases into consideration.
Many projects create a successful prototype but fail when it comes to operationalization. There are many reasons for this but the core issue is often that new optimization projects leave the cocoon of concept testing for the first time and now have to prove their worth in regular operations. In particular, the circle of users widens to include regular users in addition to the original concept team. The newcomers to the team often lack prior knowledge of the prototype. Furthermore, they do not have the time to learn the intricacies of the new methods alongside their day-to-day business.
Institutionalization: Old habits die hard
You might not consider it possible, but many projects which have successfully overcome this stage fail just before they have reached the finish line. The final, frequently under-estimated phase is that of institutionalization: The software has been launched on a technical level, but the company mentality is not able to adapt to it. The process of understanding a philosophy that is directed towards optimality starts with accepting that the mathematical method behind the new software also provides truly reliable results and is a sound basis for further decisions. The reluctance to accept new software leads to many optimization projects failing, even though all of the technical and functional obstacles have been overcome.
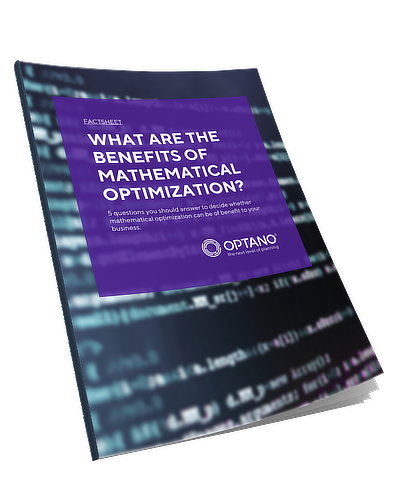
Are you interested in our factsheet?
What are the benefits of mathematical optimization?
Measures for success
The phases described above are fairly general on the one hand, on the other hand they reflect our experience of typical project development. Most projects survive the discovery phase rather well (even if this is where the first failures occur) before the stages of operationalization and institutionalization become particularly challenging. It is worth taking precautions here to ensure that you can finish the project. Without suitable counter-measures in place, these two phases are responsible for most aborted projects, both of them together account for approx. two thirds of failed projects.
In order to master these challenges, three main issues can be given consideration as early as in the planning phase:
- A clear definition of the objectives and business cases
- Paying exact attention to the integration into the productive IT infrastructure.
- Higher acceptance of mathematical optimization approaches
Fortunately, if you plan well, you can prepare for all three of the points above.
1. Defining objectives and business cases
You won’t get anywhere without a precise definition of the business scenarios. This does not only involve clearly defining the objectives which the business wants to achieve with an optimization solution. It also means drawing a clear line between closely aligned, similar objectives which are actually not a part of the project. The clearer the line between them is, the easier the later project phases will be because your arguments will be better.
Measure:
Having a small but interdisciplinary team has proven its worth. The team should be made up of specialists for important topics who can establish contacts within their respective departments when necessary. Furthermore, it is essential that management supports the defined business scenario.
2. Integration into the productive IT-Infrastructure
While creating a prototype often runs without any hitches, contact to the „real“ world consisting of IT-systems, users and, in particular, real data sources often results in numerous problems. Above all, data is often not as qualitative or as clear as was originally presumed during the creation of the prototype. This, as well as the revelation of complex special cases at a later stage often contribute to failure here.
Measure:
As important as a small amount of sample data may be, in order to manage the complexity of a new optimization problem, real data is just as indispensable. This is why real data should be included as soon as possible – perhaps even before the development of a fully-fledged optimization solution. This includes the definition of test scenarios based on this data. What should a possible solution based on this data look like? What should it certainly not look like? It is imperative that those responsible for data and who have access to such real data should be part of the team.
3. Higher acceptance
The final large hurdle is not a technical one but a question of acceptance. Nothing is as dull as tried-and-tested habits which is why timely communication is essential, and that it works in both directions.
Measure:
The optimization solution must provide understandable, proven and comprehensible added value and this should be communicated early on. Involving future users in the development phase, either as part of the inter-disciplinary team or closely aligned to it, right from the start, has stood the test of time. This facilitates not only its acceptance among many people in your company but also provides early feedback from the group of users – and that is more than its weight in gold.
More interesting articles
One last thing: Don’t forget the basics
Apart from these experiences in each specific phase, we can perceive that there are a couple of basics that should not be neglected at all. From our point of view these are indispensable fundamental virtues:
- An open, result-focused approach with the willingness to uncover a solution gradually and still want to brave the path towards it.
- An agile procedure that contains constant feedback in order to constantly question the exact requirements and be able to adjust the procedure to new insights.
Do you already know our factsheet on this topic?
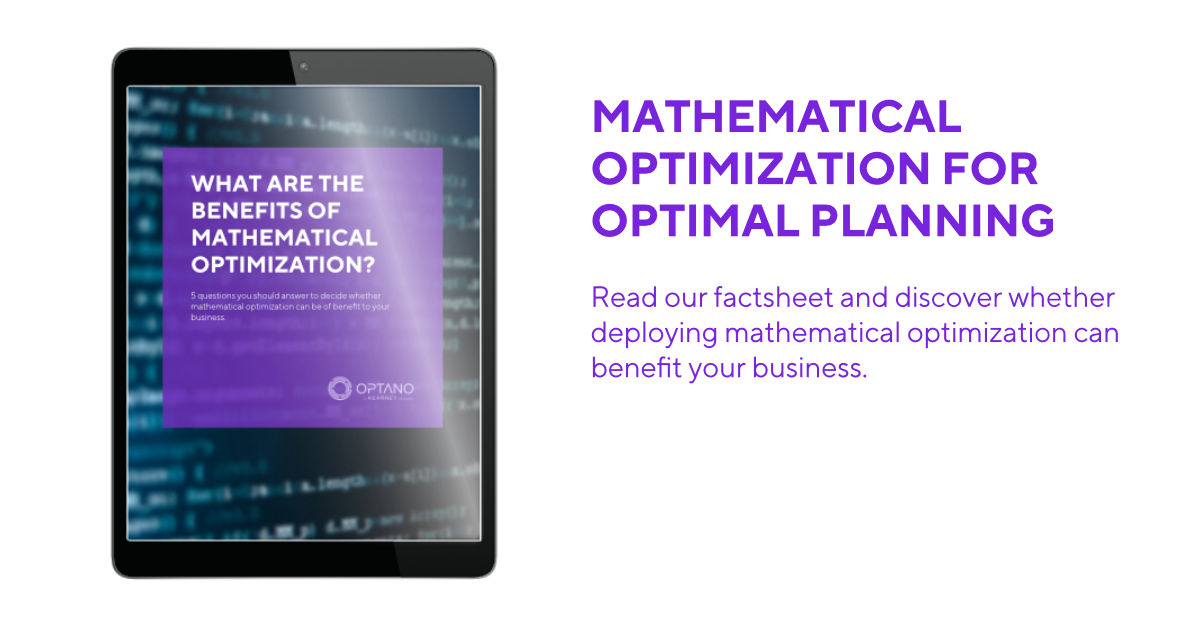
In our factsheet “What are the benefits of mathematical optimization?” we ask 5 questions to help you assess whether mathematical optimization brings benefits to your organization.
To obtain our factsheet, all you need to do is enter your contact details in the space below. A pop-up window will then open to download the whitepaper. Please note that by providing us with your email address, you agree that we may contact you on this topic. You may revoke this agreement at any time by contacting [email protected].